Life reinsurance pricing actuaries are charged with setting assumptions for reinsurance programs for our US clients. With programs today, there is a paradigm shift occurring in the industry with the new norm consisting of adding new data sources and/or the introduction of a new underwriting program, such as accelerated underwriting.
The primary challenge posed with the introduction of new data and new processes is to forecast the degree of mortality savings (or cost) from the changes being made. At SCOR our Research and Development (R&D) team does most of the research, benchmarking and analytics associated with the new norms, from designing programs that meet client objectives to measuring their impact.
A protective value study with SCOR L&H US's R&D team focused on adding instant electronic criminal history data to one of our client’s Simplified Issue programs using Velogica® (SCOR’s automated underwriting solution). A simultaneous study was done by our R&D team on the value of instant electronic clinical lab data for the same client.This triggered a reflection on the value of doing protective value studies, the success factors and their limitations.
What Is a Protective Value Study?
To start, what is a “protective value study” anyway? In its simplest form it is a determination of whether a test or tool provides (mortality) benefit in the underwriting process. The output of the study can range from a qualitative “yes” to a detailed quantitative breakdown of mortality impact by age, gender or other relevant variables.
From a direct writer perspective, the complete picture has additional considerations that may include cost of the new tool and implementation, time and cost savings from replacing other tests and potentially freeing up underwriter time.
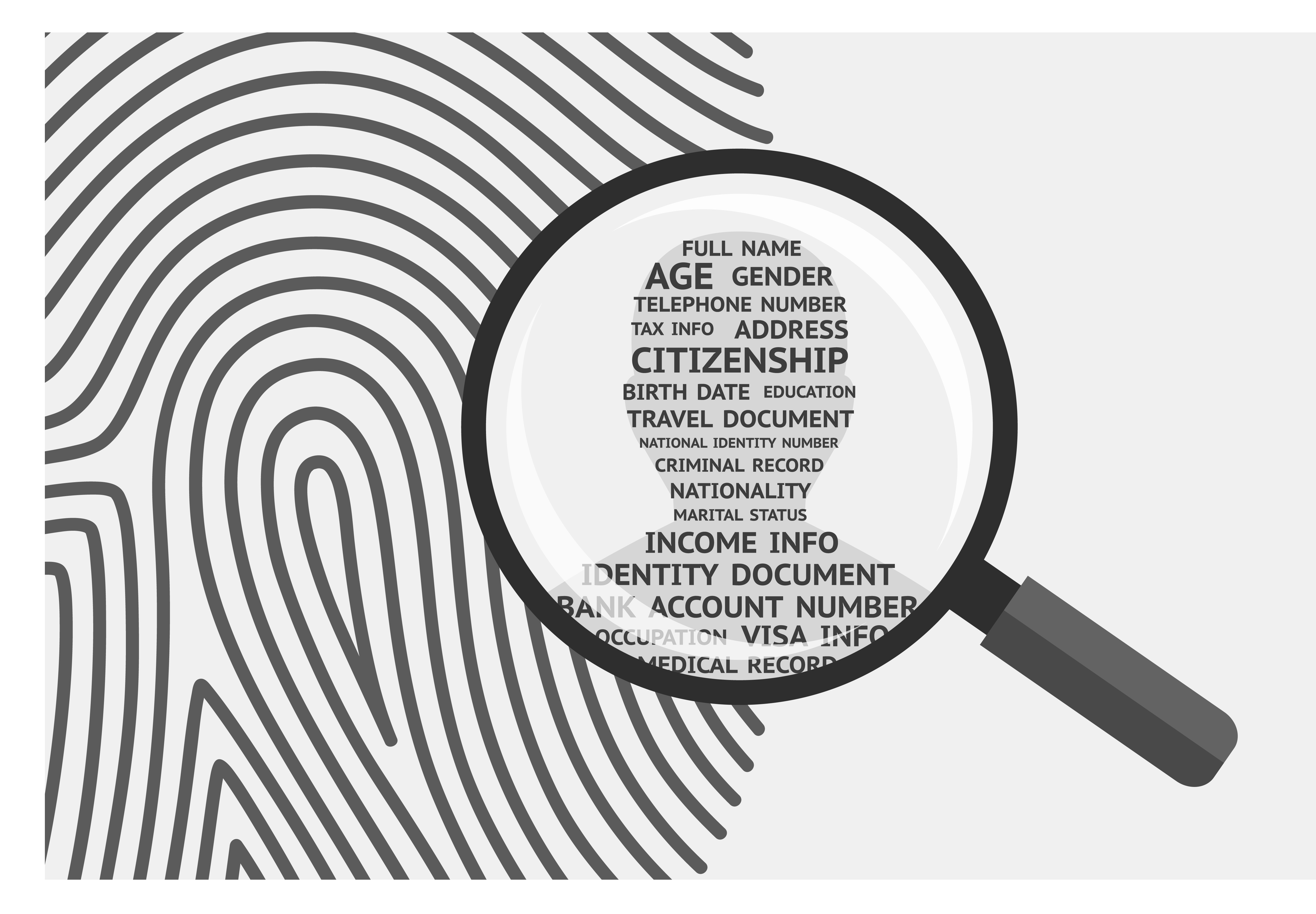
Electronic data sources allow instant verification of application responses, but how impactful is it to your business?
Why Do One?
So why do a protective value study before adopting a new data source?
- The benefits of various underwriting tools can be heavily correlated to the population to which they are being applied and to the existence of other underwriting tools. One size does not fit all in the protective value world.
- Proper stratification of risk. A study may allow you to better assign savings/costs to the correct subpopulations (age groups, gender, face amount bands, etc.)
- Personalization of use. A protective value study can be used to establish how a new data source will be used in the underwriting process based on the client’s objectives. For example, underwriting action (declination, referral, rating, approval) can be modified to hit pre-determined targets as measured by the study.
- Support discussions with your reinsurance partners when it is time to implement. Best practice is to involve your reinsurance partners early and/or collaborate with one partner for analysis and implementation.
Mortality impact is just part of the equation when evalutating a new data source or underwriting tool
What Is the Value?
Now that we understand why there is value in a personalized protective value study, how do we ensure the study produces meaningful output and direction?
- A protective value study usually has a question attached to it that drives the focus of the study.
- Data, data, data. It goes without saying that the more data that is available the better the inferences that can be made. Equally important is understanding the limitations of the new data source. For example, the criminal history data source reviewed in the study did not have coverage across all 50 states. Being aware of this fact, the SCOR Velogica® team reviewed the hit rates by issue state with the context of knowing which states may have lower or no hits due to lack of coverage.
The initial effort to scrub and integrate a new data source should not be underestimated. For example, the mapping process for electronic clinical labs data that can contain a plethora of medical codes. SCOR’s Velogica® team was well prepared to handle both sets of data preparation.
- Next, underwriting expertise! Sorry, actuaries, but before you can start crunching those numbers, underwriting expertise is needed to confirm how the data source will be incorporated in the underwriting process. For example, can this source produce immediate declines or table ratings, or will it trigger referrals in a triage process?
- The “retro-study” – the core of most protective value studies at least for actuaries – quantifies the underwriting impact on a block of business if the proposed data source was used. Usually this is accomplished by re-underwriting a block of previously submitted applications – a task that is substantially easier with an automated platform. Once the rules are established, several thousand cases can be re-underwritten within an hour.
- And yes, actuarial expertise is required to opine on assumption inputs or analyze mortality experience to quantify the impact of the re-underwriting.There are generally two broad approaches to quantification – a relative mortality impact or a full mortality study including the new data source.
The latter requires a substantial amount of claims experience and the ability to reflect the new data source for the entire mortality study population. As such, the latter approach, while more insightful, is less common.
- Finally, a good protective value study does not just provide numerical output but also contains meaningful insights:
- With a parallel clinical lab study led by R&D, the latent value in electronic clinical lab hits containing clean records—and not just the instant identification of undisclosed medical history—became apparent.
- The clinical lab study also quantified expected savings from a possible reduction in APS orders.
- In the criminal history study the low hit rate but relatively large protective value to the client from this data source was eye-opening.
Congratulations! You’ve completed your protective value study, you’ve analyzed the cost/benefit and you’re ready to go … or are you?
Yes, you probably are, but there are a few considerations to keep on the radar. The key consideration is how long are the results valid and what adjustments should be made along the way. Post-implementation monitoring is therefore critical. Consider the following situations:
- Does the addition of another data source later change the initial estimated savings? Inadvertently assuming that all changes are additive does not account for overlap and correlation. A new protective value study incorporating all the changes
should mitigate this overestimation risk.
- Does a change in the hit rate of a data source indicate a change in the protective value?
- If criminal history data is implemented and as part of the post-implementation monitoring we observe a drop in criminal history hits, does that mean we lost value or could there now be a sentinel effect that deters certain applicants?
- What if we saw the opposite, an increase in hit rate after implementation? An interesting observation in this criminal history study was that the age group with the largest hit rate was not the age group with the largest expected savings. Changes in hit rates for this client will need to be reviewed in terms of demographic shifts and changes in the underlying types of hits such as felony versus non-felony hits.
- Finally, there are aspects of new data sources that are not easily quantified in advance such as how actual placement rates will materialize.
After co-leading this protective value study, I have a renewed appreciation for how the use of an automated underwriting platform can streamline the process of analyzing and implementing a new data source and how it will become easier to tackle post-implementation considerations.
The other affirmation is the level of expertise and collaboration with the client that goes into the proper analysis of a new data source. Data, automation, analytics, expert judgement and partnership are all key ingredients in a process that aims to extract value at the speed of logic.
Special thanks to Cindy Mitchell for her underwriting input on this article and to Alex Kendrew for his actuarial modeling support in these studies.